TF-IDF(Term Frequency-Inverse Document Frequency, 词频-逆文件频率)
是一种用于信息检索与文本挖掘的常用加权技术。tf-idf是一种统计方法,用以评估一字词对于一个文件集或一个语料库中的其中一份文件的重要程度。字词的重要性随着它在文件中出现的次数成正比增加,但同时会随着它在语料库中出现的频率成反比下降。tf-idf加权的各种形式常被搜索引擎应用,作为文件与用户查询之间相关程度的度量或评级。除了tf-idf以外,互联网上的搜索引擎还会使用基于链接分析的评级方法,以确定文件在搜索结果中出现的顺序。
计算tf-idf的方式很多,如果我们用spark计算也会有现成的工具包来实现,但是对于很多sql boys 或者sql girls 来说,可能更习惯于用sql语句来实现这个值的计算。此篇文章我们抛开其它的编程语言,就用hive sql来计算语料库中所有词的tf-idf,并取得一篇文章(document)的关键词(keywords)。
1、基本公式
我们不再对每个统计值的定义和含义做过多的解释,很多文章中都会介绍到,我们只贴出基础的公式。

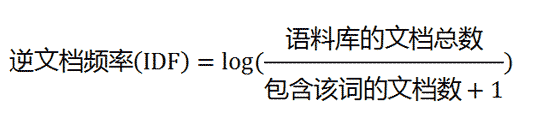

2、实现逻辑及流程
第一步,先把可能用到的中间表建出来,我们的建表没有业务硬要求,也没有特定的特定的模式,只是根据我们的公式分成了三张表,来逐步体现公式内容。第一张表示构建了所有词的基础表,为的是确定每一个词的id; 第二张表是建立一张每一个词和文章的关联表,目的是把词转化成id;第三张表是集合了所有计算所需要用的统计值。
-- 构建关键词字典表 create table if not exists key_word_dict(id int , word string) comment '关键词字典表'; -- 构建文章和词的关联表 create table if not exists article_word_dict(word_id int, article_id int) comment '文章和词的关联表'; -- tf_idf词频-逆文档频率基础表 create table if not exists article_word_tf_idf ( article_id int comment '文章id' ,article_total_word_cnt int comment '文章总词数' ,word_id int comment '词id' ,word_in_article_cnt int comment '文章中出现词的次数' ,cnt_article_with_word int comment '出现该词的文章数' ,total_article int comment '语料文章总数' ,tf double comment '词频' ,idf double comment '逆文档频率' ,tf_idf double ) comment 'tf_idf词频-逆文档频率基础表';
第二步,对每一张表的内容开始初始化或者生成对应的统计值。词的基础表我们是从原始表中的整合汇总出来的词表,并且对一些不规则的词做了过滤清洗的处理。
-- 初始化词的基础表数据 insert overwrite table key_word_dict select row_number()over() as id, a.keyword as word from ( select trim(kws) as keyword from ods_article_search fcj left join ods_article_detail detail on fcj.article_id = detail.article_id lateral view explode(split(detail.keywords, ',')) ws as kws where detail.article_id is not null group by kws ) a WHERE trim(a.keyword) IS NOT NULL AND trim(a.keyword) != "" AND trim(a.keyword) != "null" AND length(trim(a.keyword)) > 1 AND length(trim(a.keyword)) < 10 AND (trim(a.keyword) NOT REGEXP ('\”')) AND (trim(a.keyword) NOT REGEXP ('=')) AND (trim(a.keyword) NOT REGEXP ('-')) AND (trim(a.keyword) NOT REGEXP (',')) AND (trim(a.keyword) NOT REGEXP ("\"")) AND (trim(a.keyword) NOT REGEXP (" ")) AND (trim(a.keyword) NOT REGEXP ("、")) AND (trim(a.keyword) NOT REGEXP (":")) AND (trim(a.keyword) NOT REGEXP (":")) AND (trim(a.keyword) NOT REGEXP ("\\.")) AND (trim(a.keyword) NOT REGEXP ("\/")) AND (trim(a.keyword) NOT REGEXP ("?")) AND (trim(a.keyword) NOT REGEXP ("。")) AND (trim(a.keyword) NOT REGEXP ("\\(")) AND (trim(a.keyword) NOT REGEXP ("\\?")) AND (trim(a.keyword) NOT REGEXP ("!")) AND (trim(a.keyword) NOT REGEXP ("\\%")) AND (trim(a.keyword) NOT REGEXP ("\\.")) AND (trim(a.keyword) NOT REGEXP ("\\~")) AND (trim(a.keyword) NOT REGEXP ("^[0-9]+$")) AND (trim(a.keyword) NOT REGEXP ("[0-9]+$")) AND (trim(a.keyword) NOT REGEXP ("^[0-9]+")) ;
从分词表中拿到每一篇文章和分词后每个词与其的对应关系,分词表是我们之前就做好。而分词的方式我们可以通过之前的文章所介绍的利用spark + ansj来实现。
-- 生成文章和词关联的表 insert overwrite table article_word_dict select dict.id ,a.article_id from key_word_dict dict left join ( select fcj.article_id ,trim(kws) as keyword from ods_article_search fcj left join dwd_article_key_words detail on fcj.article_id = detail.article_id lateral view explode(words) ws as kws where detail.article_id is not null ) a on dict.word = a.keyword where a.keyword is not null and a.article_id is not null ;
-- 生成tf-idf基础表数据 insert overwrite table article_word_tf_idf select e.article_id ,e.article_total_word_cnt ,e.word_id ,e.word_in_article_cnt ,f.cnt_article_with_word ,f.total_article ,e.tf ,f.idf ,e.tf * f.idf as tf_idf from ( select b.article_id ,b.article_total_word_cnt ,a.word_id ,a.word_in_article_cnt ,a.word_in_article_cnt / b.article_total_word_cnt as tf from ( select sum(1) article_total_word_cnt ,article_id from article_word_dict group by article_id ) b left join ( select count(word_id) word_in_article_cnt ,article_id ,word_id from article_word_dict group by article_id ,word_id ) a on b.article_id = a.article_id where a.article_id is not null and a.article_id is not null ) e left join ( select d.word_id ,log(d.total_article / ( d.cnt_article_with_word +1)) as idf ,d.total_article ,d.cnt_article_with_word from ( select c.word_id ,c.cnt_article_with_word ,( select count(distinct article_id) as article_cnt from article_word_dict ) as total_article from ( select count(distinct article_id) as cnt_article_with_word ,word_id from article_word_dict group by word_id ) c ) d ) f on e.word_id = f.word_id where f.word_id is not null and e.word_id is not null ;
最后,我们通过tf-idf的基础表可以取一篇文章的前5个关键词,会用到hive sql的 rank over 开窗函数的组合。基本的展示效果如下图
select * from ( select a.article_id ,a.tf_idf ,b.word ,rank()over(partition by a.article_id order by a.tf_idf desc ) as sort_num from article_word_tf_idf a left join key_word_dict b on a.word_id = b.id where b.id is not null ) a where a.sort_num <= 5 ;
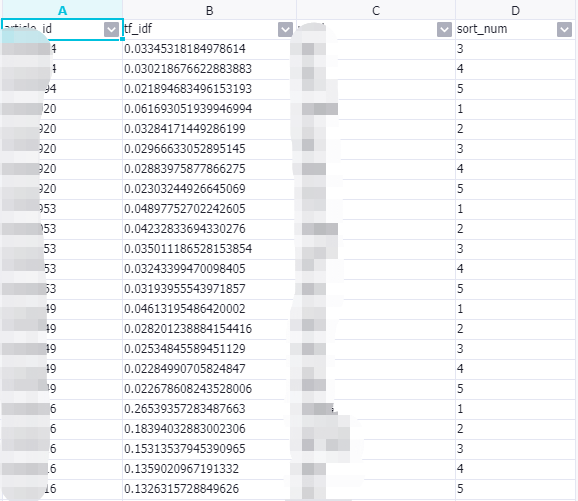